
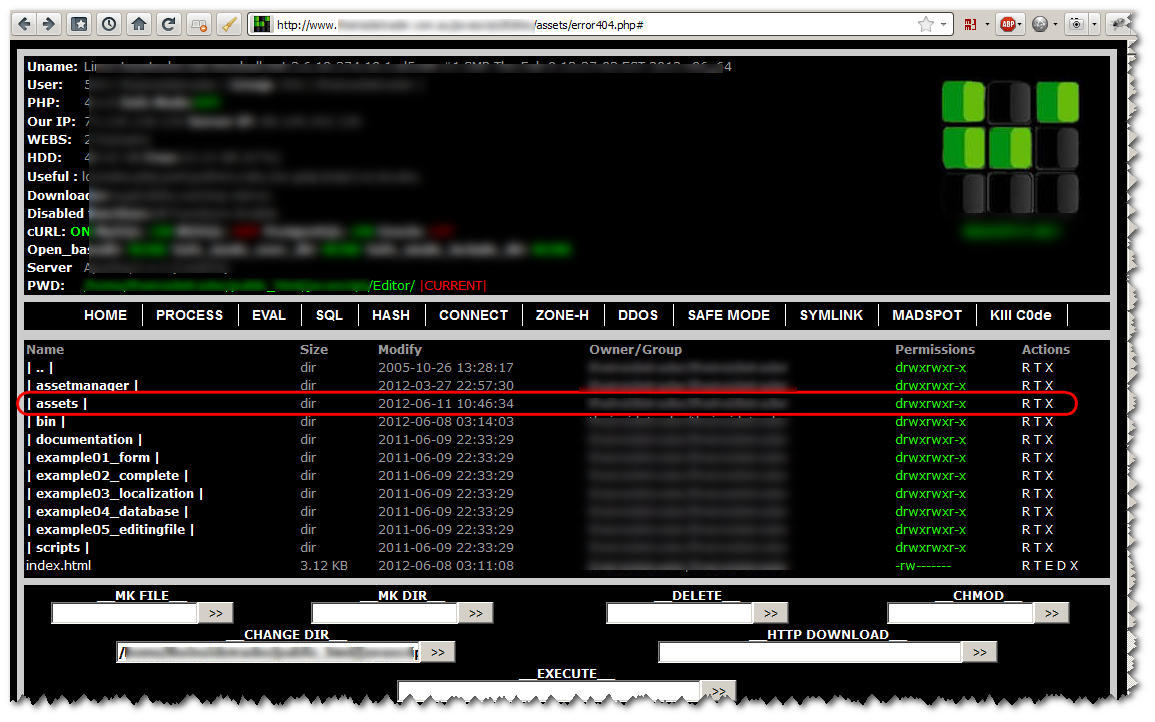
This architecture can speed up AI processing by not moving data in and out of memory. However, it also limits accuracy, which could be acceptable for some business use cases but not all.Īnother approach that is already in use in other technology spaces is in-memory computing. Research done at MIT and IBM shows that you can reduce the resources needed for running a neural network on large data sets with this approach. Sampling a pipelining, for instance, can speed up deep learning by reducing the amount of data processed. If the demand for resources to run AI systems will lead to higher computing costs and carbon output, what can we do? The answer is perhaps in finding more efficient ways for AI to utilize resources, such as processing, networking, and storage. It’s no secret that generative AI systems will displace many information workers soon. In many cases, they may try to save money within the business, perhaps by reducing the number of employees in order to offset the cost of AI systems. Businesses may find that these AI systems are not optional and are needed to drive certain critical business processes. However, higher cloud computing costs may not have the same effect on enterprises. As a result, we normally curtail usage, running the air conditioning at 74 degrees rather than 68 in the summer. Public cloud providers are like any other utility resource provider and will increase prices as demand rises, much like we see household power bills go up seasonally (also based on demand). This demand will most likely be met with more data centers housing power-hungry servers and networking equipment. More demand for AI means more demand for the resources these AI systems use, such as public clouds and the services they provide. This surge will be led by businesses that are looking for an innovative advantage, such as intelligent supply chains, or even thousands of college students wanting a generative AI system to write their term papers. Demand will skyrocket for AI services, such as the generative AI systems that are driving interest now as well as other AI and machine learning systems. You really don’t need to do much research to predict what’s going to happen here. I would say that now they are tightly coupled. In fact, the rise of cloud, as you may have guessed, was roughly aligned with the rise of AI in the past 10 to 15 years. What once was unapproachable is now cost-efficient enough to be possible with public clouds.
